What Is OSINT In Cybersecurity?
OSINT (Open Source Intelligence) is a pivotal concept within the realm of cybersecurity. It denotes the procedure of collecting and analyzing data acquired from public sources. Unlike covert intelligence methods, OSINT harnesses openly accessible data. Here’s an in-depth look at OSINT and its role in cybersecurity:
1. OSINT Sources
OSINT in cybersecurity involves extracting information from a plethora of sources, such as:
- News agencies and digital publications.
- Government databases and public records.
- Social media channels and online forums.
- Scholarly articles and academic databases.
- Multimedia content like videos and podcasts.
- Geospatial tools and satellite images.
- Website data and domain registries.
2. OSINT Applications in Cybersecurity
- Defensive Measures: National defense bodies and governments employ OSINT (Open Source Intelligence) to track potential security threats, evaluate global political shifts, and understand opponents’ capabilities.
- Business Intelligence: Firms leverage OSINT for competitive insights, market evaluations, risk determinations, and thorough vetting processes.
- Cybersecurity Analysis: Professionals in cybersecurity use OSINT methodologies to identify vulnerabilities, carry out threat evaluations, and discern the tactics and strategies of malicious entities.
- Investigative Reporting: OSINT aids journalists in confirming stories, probing into leads, and assembling evidence for disclosures.
3. Benefits of OSINT in Cybersecurity
- Ease of Access: The foundational premise of OSINT in cybersecurity is tapping into publicly dispensed data, making it a universally accessible intelligence model.
- Economical: Leveraging public sources, OSINT operations can be executed with nominal financial investments.
- Varied Viewpoints: The diverse nature of OSINT sources offers a multifaceted perspective, paving the way for a holistic comprehension of cybersecurity scenarios.
4. OSINT Challenges in Cybersecurity
- Data Deluge: With the vastness of available data, sifting through to pinpoint relevant information becomes a challenge. Hence, potent analysis tools are indispensable in cybersecurity.
- Validity and Cross-checking: Given that public data can sometimes be slanted, outdated, or intentionally misleading, OSINT in cybersecurity mandates rigorous validation and multi-source referencing.
- Ethical Implications: Ethical dilemmas emerge when using public data, especially when individuals remain oblivious to their data serving intelligence purposes.
To encapsulate, OSINT (Open Source Intelligence) stands as a beacon in the cybersecurity landscape, offering invaluable insights from open data. However, effective OSINT in cybersecurity demands meticulous analysis, rigorous verification, and an unwavering commitment to ethical standards.
Introduction
Understanding and interpreting human emotions is a complex task that has fascinated researchers and scientists for decades. The ability to accurately detect and analyze a person’s emotions can have numerous applications, ranging from improving mental health support to enhancing user experiences in various industries.
In recent years, significant progress has been made in the field of emotion detection, with one approach known as OSINT gaining attention for its effectiveness in uncovering individual moods.
OSINT, an acronym for “Observing Sentiments through Intelligent Networks,” refers to a collection of methodologies and techniques that leverage cutting-edge technologies, such as machine learning, natural language processing, and facial recognition, to discern and interpret the emotions of individuals.
By analyzing a combination of verbal and non-verbal cues, OSINT aims to provide deep insights into a person’s emotional state, contributing to a deeper understanding of human behavior and facilitating more empathetic interactions.
One of the primary advantages of OSINT (Open Source Intelligence) is its ability to capture emotions in real-time, allowing for timely interventions or tailored responses in various domains. Whether it’s in mental health counseling, customer service, or even educational settings, the ability to accurately detect and respond to someone’s emotions can greatly enhance the effectiveness and quality of interactions.
Within the realm of OSINT, various methodologies have emerged, each with its own unique approach and strengths. These methods encompass a wide range of data sources, including text-based analysis, audio and speech processing, facial expression recognition, and physiological signals.
By combining multiple modalities, OSINT strives to create a more comprehensive understanding of an individual’s emotional state, considering both explicit and implicit cues.
In this article, we will delve into some of the most prominent methods employed within the OSINT (Open Source Intelligence) framework for detecting and analyzing emotions. We will explore the underlying principles, techniques, and challenges associated with each method.
Additionally, we will highlight practical applications and potential future developments in the field, as well as the ethical considerations surrounding the use of emotion detection technologies.
By understanding the methods used in OSINT for emotion detection, we aim to shed light on the advancements in this exciting field and emphasize the potential benefits it can bring to various sectors.
Whether you are interested in psychology, artificial intelligence, human-computer interaction, or simply curious about the intricate workings of human emotions, this article will serve as a comprehensive guide to the methodologies utilized in OSINT for uncovering and understanding individual moods.
Key Elements
Check person exists or not
In today’s digital age, where online interactions and virtual identities have become commonplace, verifying the authenticity of individuals has become an important concern. With the rise of fake profiles, identity theft, and online scams, the need for tools and techniques to check whether a person truly exists has grown exponentially.
Addressing this challenge, the open-source project “AmIReal” provides a solution to determine the authenticity of individuals and verify their existence.
AmIReal: A Tool for Verification: AmIReal, available on GitHub at https://github.com/seintpl/AmIReal/, is a powerful tool designed to check the existence of a person. Developed by a team of dedicated contributors, AmIReal leverages advanced algorithms and data analysis techniques to assess the authenticity of individuals based on available information and online footprints.
How AmIReal Works: AmIReal utilizes a combination of data mining, pattern recognition, and machine learning algorithms to analyze various data sources, including social media profiles, online presence, public records, and more. By aggregating and analyzing these data points, AmIReal generates a comprehensive assessment of the person’s existence.
Key Features of AmIReal
- Data Aggregation: AmIReal gathers information from multiple sources to build a comprehensive profile of the individual under scrutiny.
- Algorithmic Analysis: Advanced algorithms process the collected data to identify patterns, anomalies, and inconsistencies that may indicate fraudulent or non-existent profiles.
- Machine Learning Integration: AmIReal incorporates machine learning models to continuously improve its accuracy and adapt to evolving trends in online identity verification.
- Transparent and Open-Source: AmIReal is an open-source project, allowing the community to contribute, review, and enhance the tool’s capabilities over time.
- User-Friendly Interface: The tool offers an intuitive and user-friendly interface, making it accessible to both technical and non-technical users.
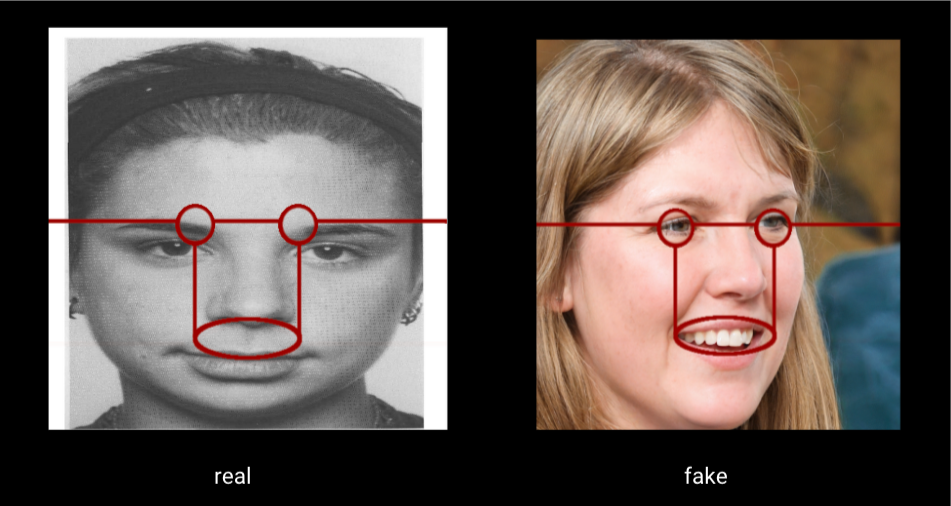
1. Check Image Moods
In the realm of human psychology and understanding, emotions play a central role in shaping our experiences and interactions. In his groundbreaking book, “Emotions Revealed,” renowned psychologist Paul Ekman takes us on a captivating journey into the depths of human emotions.
Through his extensive research and real-life anecdotes, Ekman offers profound insights into the intricacies of emotions and the ways they influence our lives.
“Emotions Revealed” serves as a comprehensive guide to decoding the vast array of human emotions. Ekman draws upon his pioneering work in facial expressions and body language to shed light on the universal nature of emotions across cultures and societies.
By dissecting various emotional states, such as happiness, anger, fear, sadness, and surprise, Ekman unravels the complex web of factors that contribute to their manifestation.
Ekman combines scientific rigor with engaging storytelling to convey his research findings effectively. Drawing from his encounters with diverse populations and even his experiences studying facial expressions in isolated tribes, Ekman presents a wealth of evidence supporting his theories.
He reveals the role of evolutionary biology, social conditioning, and individual differences in shaping emotional responses, offering readers a fascinating glimpse into the mechanisms that underpin our emotional lives.
Beyond its scientific exploration, “Emotions Revealed” equips readers with practical tools for understanding and managing their own emotions, as well as decoding the emotional cues of others. Ekman emphasizes the importance of emotional awareness and empathy in fostering healthy relationships and effective communication.
By recognizing the subtle nuances of facial expressions, body language, and vocal intonations, readers can gain valuable insights into the emotions hidden beneath the surface.
For example, Ekman highlights that disgust is a universal emotion with distinct facial expressions and physiological responses. He explores how disgust serves as a protective mechanism, signaling potential harm or contamination. By investigating the triggers and variations of disgust across cultures, Ekman uncovers the intricate relationship between personal experiences, cultural norms, and the expression of this powerful emotion.
Or In “Emotions Revealed,” Ekman delves into the realm of slight sadness, a nuanced emotional state often overshadowed by intense grief or depression. Ekman emphasizes that even subtle displays of sadness carry valuable information about a person’s emotional well-being.
By examining microexpressions and subtle behavioral cues, he unravels the hidden layers of slight sadness and its impact on human interactions and relationships.
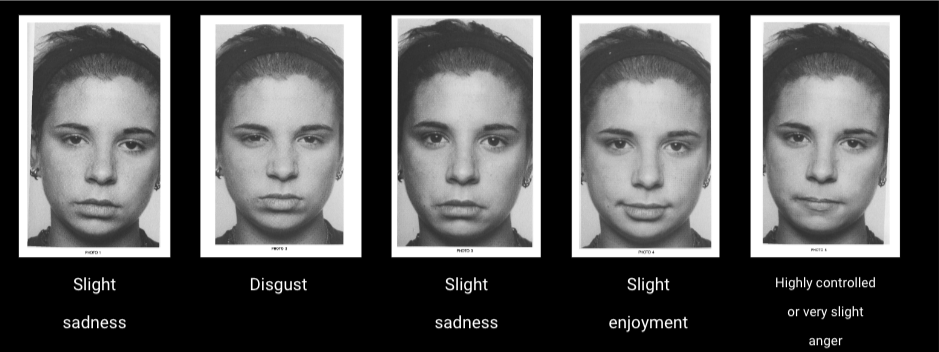
2. Automate Check Image Moods
FaceReader utilizes advanced algorithms and machine learning techniques to automatically detect and analyze facial expressions of emotions. It can analyze a wide range of emotions such as happiness, sadness, anger, surprise, fear, and disgust, among others. The software provides researchers, psychologists, and other professionals with a quantitative assessment of emotional responses.
The process typically involves the following steps:
- Data Capture: FaceReader analyzes facial expressions from video recordings or images. These visuals are usually captured through a camera or webcam.
- Facial Expression Recognition: Using sophisticated computer vision algorithms, FaceReader detects and recognizes key facial landmarks, muscle movements, and expressions.
- Emotion Analysis: Based on the detected facial expressions, FaceReader applies machine learning algorithms to classify and quantify the displayed emotions. It provides detailed reports on the intensity and duration of specific emotions.
- Data Visualization and Analysis: FaceReader generates visualizations, such as graphs and heatmaps, to represent the emotional responses over time. Researchers can use these visualizations to gain insights into emotional patterns and correlations with other variables.
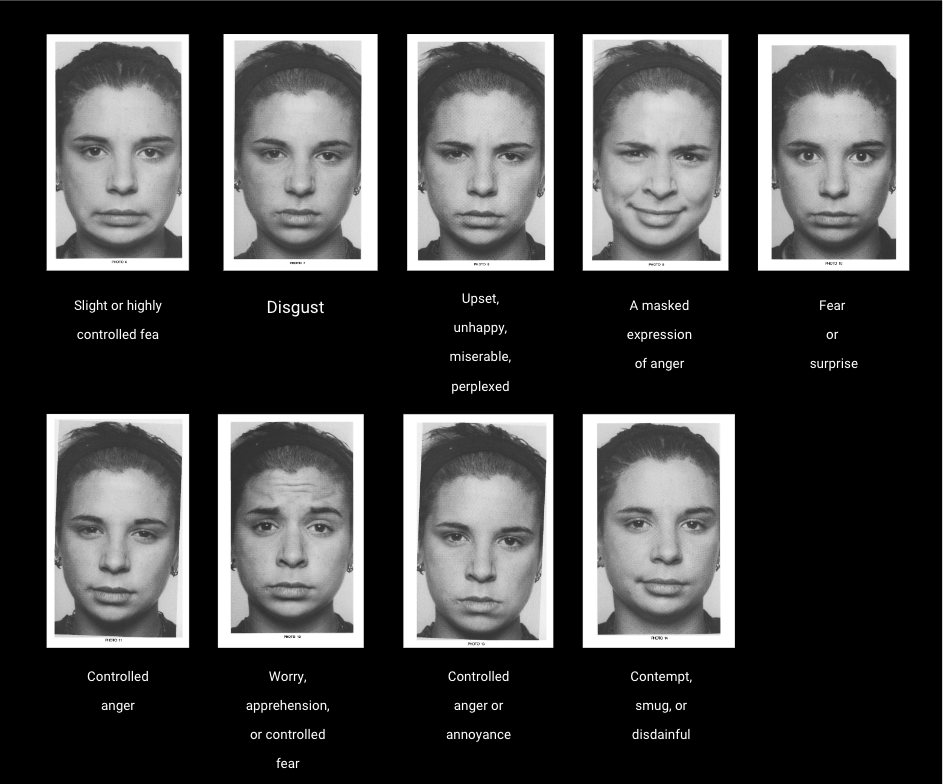
Applications of FaceReader: FaceReader finds applications in various domains, including psychology, market research, user experience studies, and human-computer interaction. Researchers and professionals use it to explore emotional responses to stimuli, evaluate product usability, assess emotional engagement in media content, and conduct emotion-related research.
https://www.noldus.com/facereader/measure-your-emotions
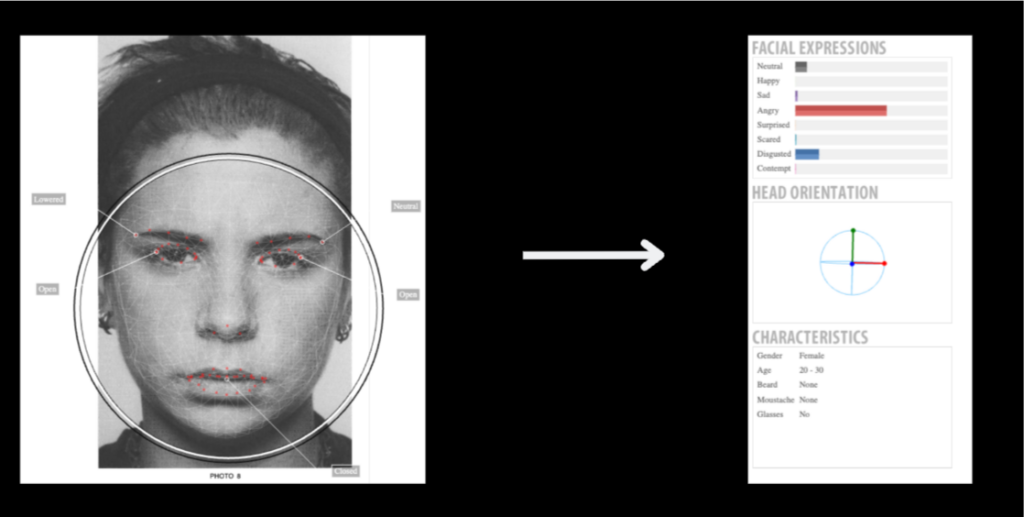
3. Automate Check Video Moods
The website “https://demo.morphcast.com/sdk-ai-demo/#/” provides a demonstration of the MorphCast AI SDK for measuring emotions in live video. MorphCast is an AI-powered software development kit that enables real-time emotion analysis and measurement using facial expressions captured from a live video feed.
Measuring Emotions in Live Video with MorphCast: The MorphCast AI SDK utilizes computer vision and machine learning techniques to analyze facial expressions and infer emotions in real time. It can detect and track facial landmarks, muscle movements, and other visual cues to assess emotional states. Here’s a general overview of how emotion measurement works using MorphCast:
- Live Video Input: MorphCast takes a live video feed as input, typically from a camera or webcam. The video feed captures the person’s facial expressions and movements.
- Facial Analysis: The AI algorithms in MorphCast analyze the video frames and extract relevant facial features, such as key landmarks, facial muscle movements, and expression dynamics.
- Emotion Recognition: Based on the extracted facial features, MorphCast applies machine learning models to recognize and classify emotions. It identifies patterns and cues associated with different emotional states, such as happiness, sadness, anger, surprise, fear, and more.
- Real-time Measurement: MorphCast provides real-time measurement and analysis of emotions. It can track the intensity and changes in emotional expressions over time, allowing for dynamic monitoring and assessment.
- Visual Feedback: The results of the emotion analysis are often visualized in real-time, providing visual feedback such as emotion labels, facial heatmaps, or overlays that highlight specific facial regions associated with different emotions.
Applications of MorphCast: The MorphCast AI SDK has various applications across industries and domains. It can be utilized in areas such as customer experience analysis, market research, user testing, mental health monitoring, virtual reality experiences, and interactive digital media. By measuring emotions in live video, MorphCast enables real-time insights into emotional engagement, user experiences, and audience responses.
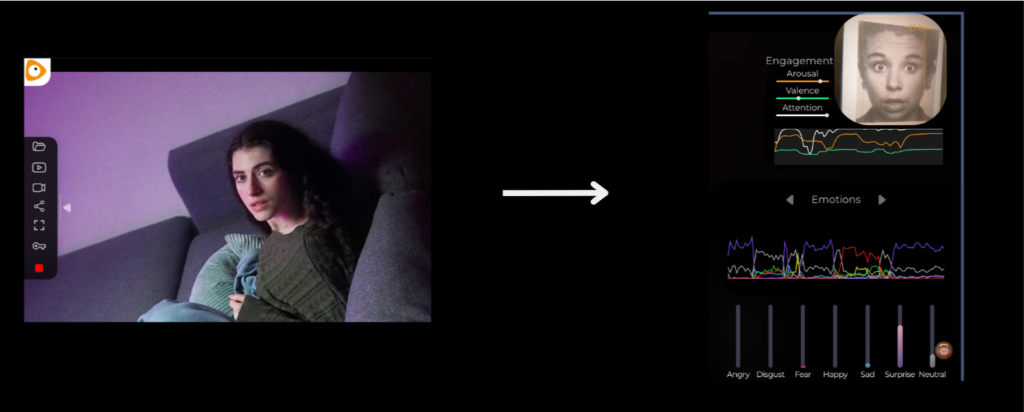
4. Find Tweets Moods
The website “https://www.csc2.ncsu.edu/faculty/healey/tweet_viz/tweet_app/” hosts a tweet visualization tool developed by Dr. Christopher Healey from North Carolina State University. The tool allows users to visualize and analyze emotions in tweets.
Measuring Emotions in Tweets with the Tweet Visualization Tool: The tweet visualization tool uses natural language processing (NLP) techniques to analyze the text content of tweets and infer the emotions expressed within them. Here’s an overview of how emotion measurement works using this tool:
- Input Tweets: Users provide a set of tweets or a specific Twitter user’s timeline as input to the tweet visualization tool. The tool processes the text content of these tweets.
- Text Analysis: The tool applies various NLP techniques to analyze the tweets’ text. This may include tokenization, part-of-speech tagging, and sentiment analysis to extract meaningful information from the text.
- Emotion Detection: Using sentiment analysis or other techniques, the tweet visualization tool identifies the emotional content within the tweets. It recognizes and assigns emotions such as happiness, sadness, anger, surprise, fear, or others based on the language used in the tweets.
- Visualization: Once the emotions in the tweets are detected, the tweet visualization tool generates visualizations to represent the emotional patterns. These visualizations may include graphs, charts, word clouds, or other graphical representations to provide insights into the emotional content of the tweets.
- Analysis and Interpretation: Users can analyze and interpret the emotional patterns found in the tweets using the generated visualizations. This can help understand the sentiment and emotional trends within the tweets, identify topics that evoke strong emotions, or detect changes in emotional expression over time.
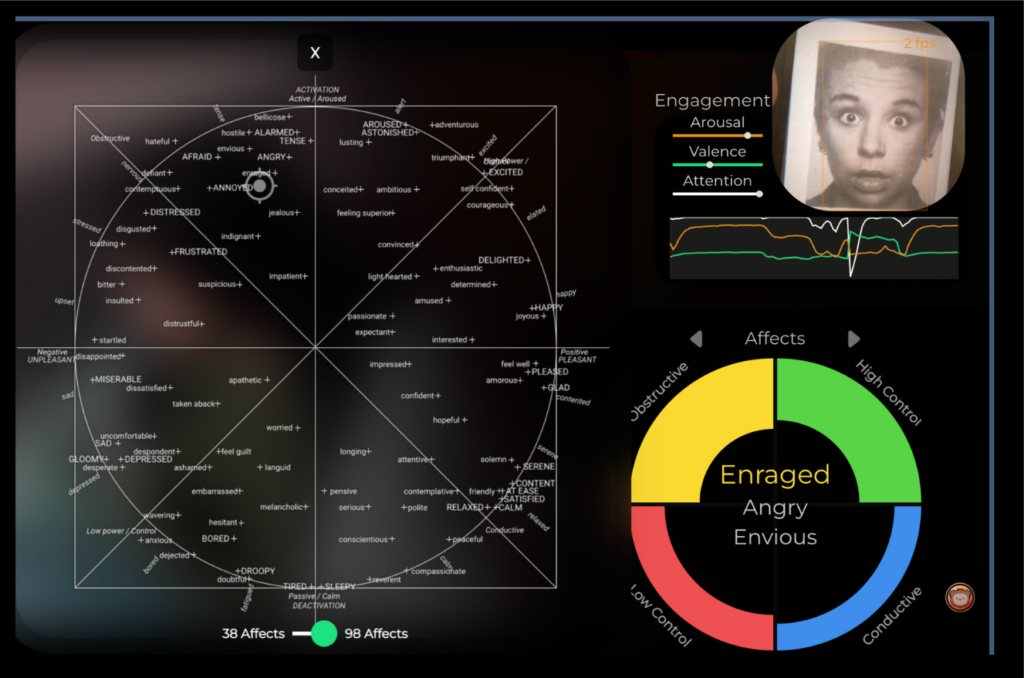
Applications of the Tweet Visualization Tool: The tweet visualization tool has various applications in social media analysis, sentiment analysis, and understanding public opinion. It can be used to study online conversations, track sentiment towards specific topics or brands, identify emotional responses to events or news, and gain insights into the collective emotions expressed on Twitter.
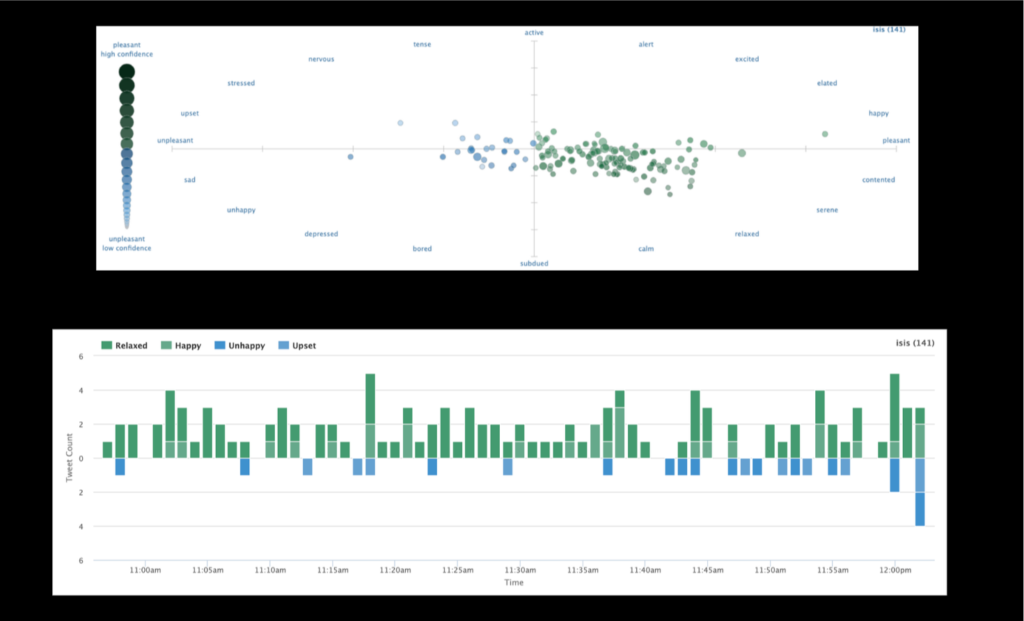
5. Find Text Moods
The OpenAI Chat API, available at “https://chat.openai.com/“, is designed to generate responses to text-based prompts. While it can provide language generation capabilities, it does not have built-in functionality specifically tailored for measuring emotions in text. However, you can utilize the API in combination with external sentiment analysis tools or techniques to assess the emotional tone of text inputs.
Here’s a general approach to measuring emotions in text using the OpenAI Chat API and external sentiment analysis:
- Text Prompt: Construct a text prompt or question that elicits a response containing emotional content. For example, you could ask about someone’s feelings, opinions, or experiences related to a specific topic.
- OpenAI Chat API: Send the constructed prompt to the OpenAI Chat API as the input. The API will generate a response based on the provided prompt.
- Response Analysis: Receive the response from the API and apply sentiment analysis techniques or tools to analyze the emotional tone of the generated text. Sentiment analysis algorithms can assign sentiment labels such as positive, negative, or neutral to text based on the words used, context, and grammatical structure.
- Emotion Measurement: Convert sentiment analysis results into emotion measurement by mapping sentiment labels to corresponding emotional states. For example, positive sentiment could be associated with emotions like happiness or excitement, while negative sentiment could be linked to emotions like sadness or anger.
- Interpretation and Evaluation: Interpret the emotional measurement results and evaluate the emotional content of the generated text. Consider the nuances and potential limitations of sentiment analysis, such as the influence of sarcasm, cultural differences, or the inability to capture complex emotions accurately.
By combining the OpenAI Chat API with external sentiment analysis techniques or tools, you can gain insights into the emotional content of the generated text. It’s important to note that the accuracy and effectiveness of sentiment analysis can vary depending on the quality of the sentiment analysis algorithm or tool used and the characteristics of the text being analyzed.
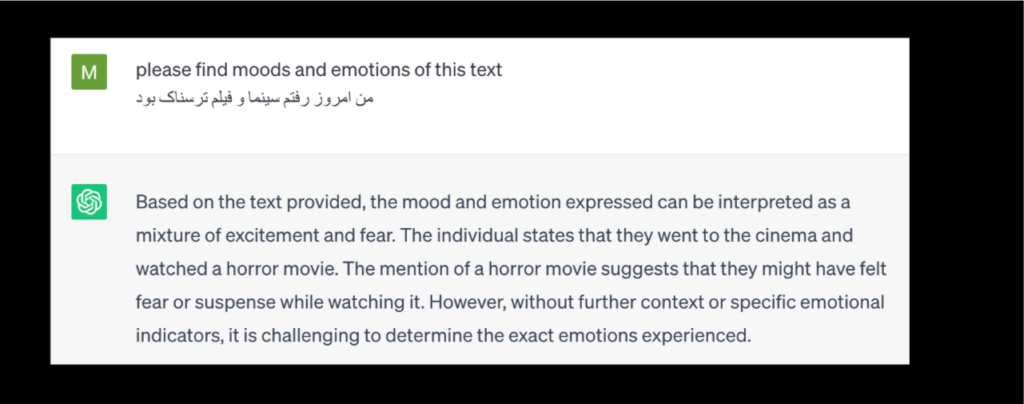
#1 Find Relation Moods:
- Install Neo4j: Start by installing and setting up Neo4j, which is a graph database management system. You can download and install Neo4j from the official website (https://neo4j.com/). Follow the installation instructions provided for your specific operating system.
- Set up the Neo4j Database: Once Neo4j is installed, you need to set up a database to store your data. Launch the Neo4j browser and create a new database. You can define the schema and configure the database settings according to your requirements.
- Gather Mood Data: Use Selenium, which is a web automation tool, to scrape or collect mood-related data from websites or other online sources. Selenium can interact with web pages, navigate through them, and extract the desired information. You would typically write Python code using the Selenium library to automate web scraping tasks.
- Store Mood Data in Neo4j: With the mood data obtained through Selenium, establish a connection to your Neo4j database using a Neo4j driver for your chosen programming language (e.g., Python). Write code to insert the mood data into the database. Define appropriate node and relationship structures in the database schema to represent moods and their relations.
- Define Mood Relations: Determine the relationships between different moods based on your analysis or desired criteria. For example, you might establish relationships based on similarities, contrasts, or causality between moods. Define and create these relationships in the Neo4j database using the appropriate queries or commands provided by the Neo4j driver.
- Query and Analyze the Mood Relations: Utilize Neo4j’s query language, Cypher, to perform queries on the database and retrieve information about mood relations. You can execute Cypher queries using the Neo4j driver in your chosen programming language. Analyze the results and extract insights from the mood-related data stored in Neo4j.
By combining the web scraping capabilities of Selenium with the graph database features of Neo4j, you can collect mood data from online sources and establish relationships between different moods. This approach allows you to store, query, and analyze the mood-related information in a graph database, enabling you to identify patterns and connections between various moods.
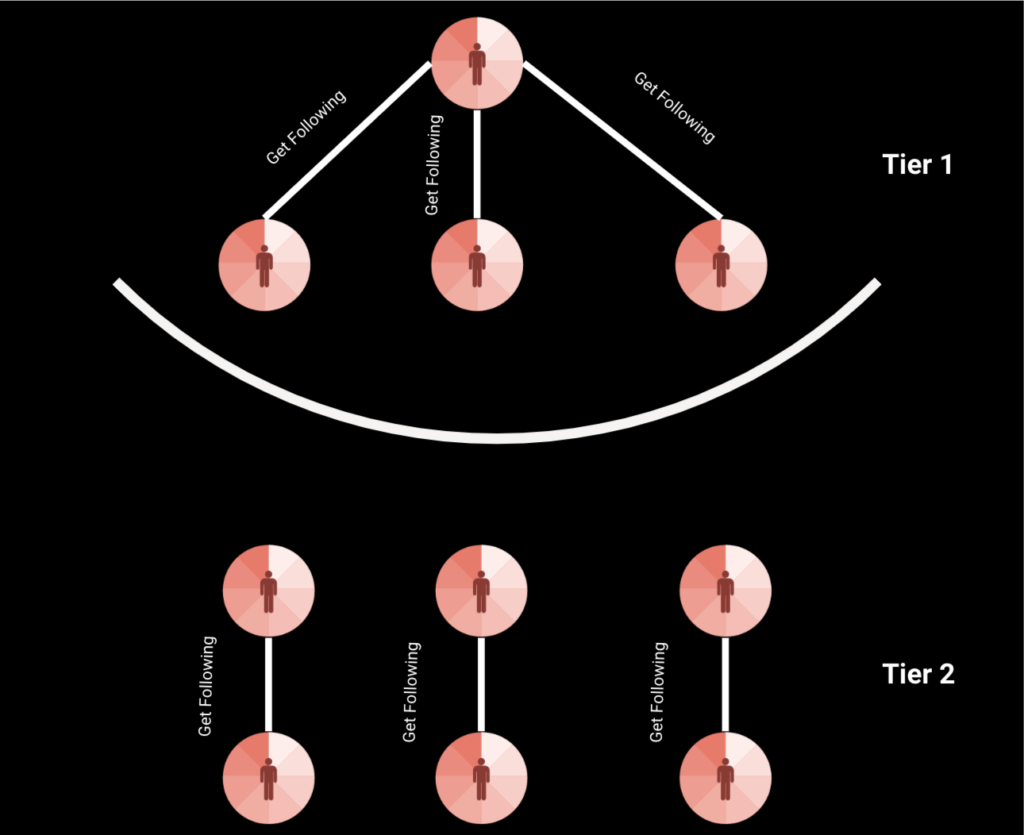
Train Characterize Bot Generation
To train a character bot generation model to generate prompts for different moods using the websites “https://poe.com/” or “https://beta.character.ai/“, you would typically follow these steps:
- Data Collection: Gather a diverse set of text data that represents different moods. You can collect text samples from various sources such as literature, movies, social media, or online forums. Ensure that the collected data covers a wide range of emotions and moods.
- Data Preprocessing: Clean and preprocess the collected text data to remove any irrelevant or noisy information. Perform tasks such as removing special characters, tokenizing the text into words or sentences, and converting the text to a suitable format for training.
- Model Selection: Choose a suitable language model or deep learning framework for training your character bot generation model. Options can include GPT (such as GPT-3 or GPT-4) from OpenAI or other similar models available in the market. Consider the specific requirements of your project, such as model size, capabilities, and available resources.
- Model Training: Fine-tune or train the selected language model using the preprocessed text data. Fine-tuning involves feeding the data into the language model and adjusting its parameters to learn the patterns and structures of the input text. This process typically requires significant computational resources.
- Prompt Generation: Develop a system that takes a specific mood as input and generates appropriate prompts using the trained language model. The prompts should be tailored to elicit responses that align with the desired mood. For example, you can provide the character bot with different starting phrases or specific instructions to set the tone or emotion for the generated response.
- Evaluation and Iteration: Evaluate the quality and coherence of the generated prompts and corresponding bot responses. Iteratively refine and improve the training process, fine-tuning parameters, or adjusting the data to enhance the model’s ability to generate relevant and contextually appropriate prompts for different moods.
- Integration with Website: Integrate the trained character bot generation model into the desired website, whether it’s “https://poe.com/” or “https://beta.character.ai/“. This may involve leveraging APIs or SDKs provided by the platforms to enable seamless interaction between users and the character bot.
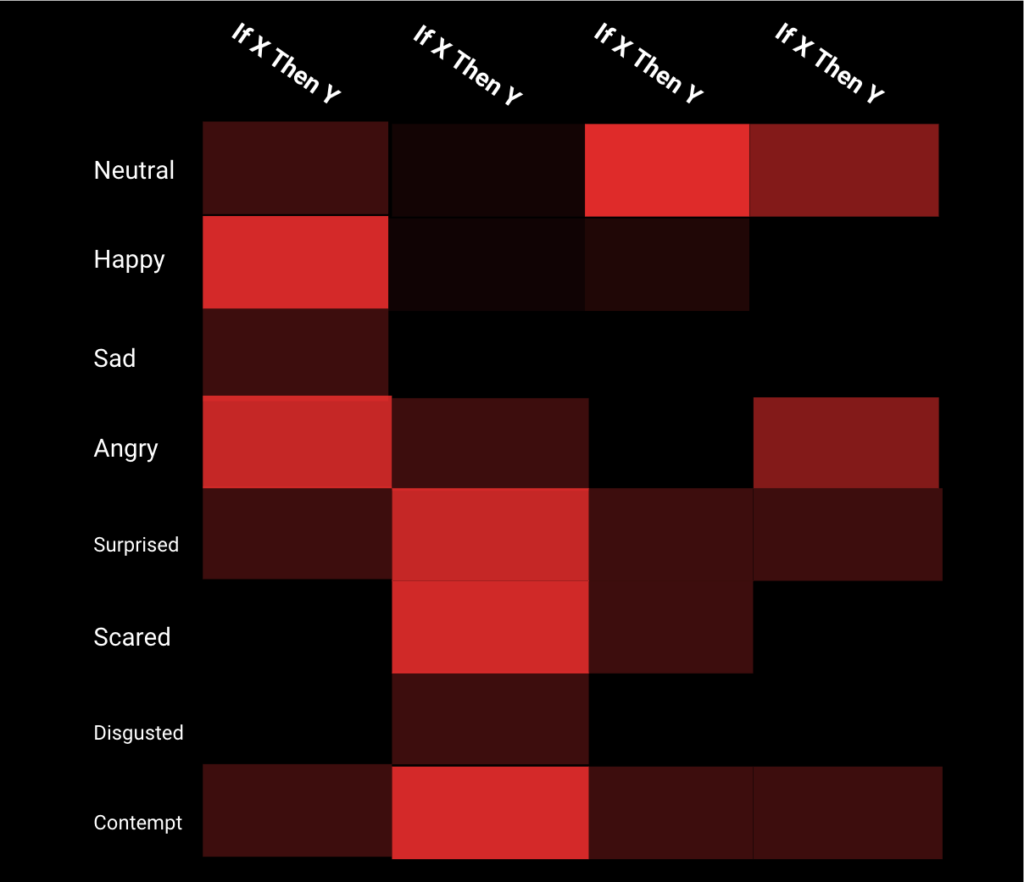
Trained Prompt
- Explain the communication style and tone used by the bot.
- Discuss the bot’s level of responsiveness and engagement.
- Analyze the bot’s ability to understand and respond appropriately to user queries.
- Assess the bot’s level of empathy and emotional intelligence in interactions.
- Provide examples of how the bot interacts with users.
- Evaluate the bot’s consistency in providing accurate and reliable information.
- Explore the bot’s adaptability to different user preferences and needs.
- Describe any user feedback or testimonials regarding the bot’s characterization.
- Evaluate the bot’s overall effectiveness in achieving its intended purpose.
Warning
- Ethical concerns: AI systems may have access to personal information and emotions, which raises privacy and security concerns. It is crucial to handle sensitive emotional data responsibly and ensure it is not misused or exploited.
- Emotional manipulation: AI systems designed to understand and respond to human emotions may have the potential to manipulate or exploit individuals’ feelings for personal gain or malicious purposes. Safeguards must be in place to prevent such manipulation.
- Lack of empathy: While AI can analyze and mimic human emotions to some extent, it does not possess genuine emotions or empathy. Relying solely on AI for emotional support or guidance may result in a lack of understanding and true human connection.
- Cultural biases: AI algorithms trained on specific datasets can inherit biases, including those related to emotions and cultural contexts. This can lead to inaccurate or inappropriate responses, reinforcing stereotypes or discriminating against certain individuals or groups.
- Oversimplification of emotions: Human emotions are complex and multifaceted, influenced by various factors such as personal experiences, culture, and context. AI systems may oversimplify or generalize emotions, leading to misinterpretations or inadequate responses.
- Emotional misreading: AI systems attempting to recognize and respond to emotions may make errors in identifying or understanding human emotional states accurately. Relying on these systems for critical decisions or emotional support without human oversight can lead to unintended consequences.
- Lack of human judgment: AI systems lack the ability to exercise human judgment and intuition, which is crucial for interpreting complex emotional situations and providing appropriate responses. Human involvement and oversight are essential to avoid potential pitfalls.
- Emotional dependency: Overreliance on AI for emotional support or decision-making may lead to a loss of human-to-human interaction, potentially impacting mental and emotional well-being. It is important to maintain a healthy balance between AI and genuine human connections.
- Unintended emotional biases: AI algorithms trained on historical data may inadvertently perpetuate emotional biases or reinforce negative emotional patterns. Regular monitoring and reevaluation of AI models are necessary to mitigate the risk of perpetuating harmful emotional biases.
- Emotional privacy concerns: Sharing personal emotions and experiences with AI systems raises concerns about the confidentiality and security of such information. It is important to ensure that emotional data is protected and handled in accordance with privacy regulations and best practices.
Special Thanks to @𝕊𝕖𝕔𝕥𝕠𝕣𝟘𝟛𝟝(https://sector035.nl/)